Improving Product Gap & Revenue Analytics with Generative AI for PresKale
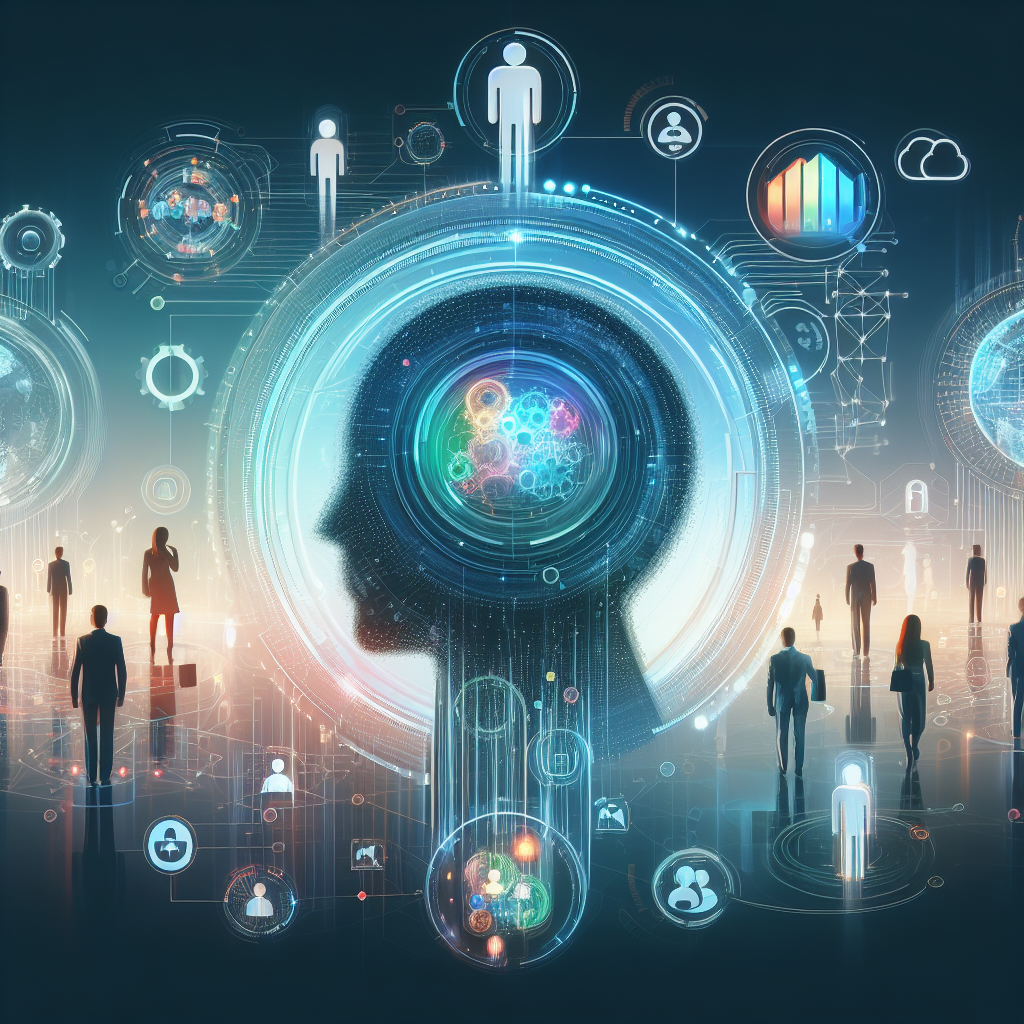
About Customer
PreSkale is a AI-driven PreSales platform to improve Technical Sales wins. The platform integrates with CRM, calendar, and product tools, automating activity tracking for efficiency. It unifies product gap management, streamlining the identification and resolution of gaps to revive blocked deals. With advanced analytics, PreSkale delivers utilization reports, and product revenue insights, enabling PreSales to influence revenue growth.
Objective
Preskale, a leading technology company, aims to enhance its product gap analysis tool to streamline the identification of critical product analytics and their revenue impact for their customers. Currently, the process is manual and time-consuming so PreSkale sought to leverage ShellKode's GenAI solutions to further enhance their efficiency and scale up their capabilities.
ShellKode Solution
ShellKode built a Bedrock-based Generative AI solution that analyzes and prioritizes product features based on their potential revenue impact stored in Jira tickets. This ensures a data-driven approach to feature prioritization and automatically maps identified product gaps to their potential revenue impact, enhancing precision and minimizing subjective interpretation
- Data Collection and Storage: Jira ticket data, including ticket ID, description, and title, is collected and stored in an Amazon S3 bucket in CSV format, ensuring centralized and accessible data.
- Contextualization with Amazon Bedrock LLM Chain: Leveraging the power of Amazon Bedrock, our solution employs a Language Model (LLM) chain to process the data. The Bedrock LLM chain defines ticket contexts and generates tags for each ticket based on the provided product description and title.
- Tag-based Ticket Group Classification: The ticket ID and generated tags are then seamlessly transferred to the second LLM chain. This chain classifies ticket groups based on the generated tags, providing a systematic and automated approach to categorizing and organizing the vast ticket data.
- Insights Generation for Product Analytics: The classified ticket groups and associated tags are harnessed by the customer's platform to generate valuable insights. This enables our clients to pinpoint areas of improvement, enhance product development strategies, and prioritize resources effectively.
Outcome
- Automated contextual data capture to deliver a 40% improvement in product Gap creation.
- Improved data analytics with the data captured from automation.
- Automating tag-based ticket group classification resulted in a 25% reduction in manual effort.
- Elevated customer satisfaction by providing intelligent suggestions with Gen AI.